I am now a first-year PhD candidate at Centre for Language Studies (CLS) , Radboud University, supervised by M.A. Larson (Martha), co-supervised by C. Tejedor Garcia (Cristian), and L.F.M. ten Bosch (Louis). Before that, I was a visiting researcher at the Audio Information Research(AIR) Lab of University of Rochester. I used to be a senior researcher at Tencent AI Lab, AI-Generated Content (AIGC) Center for speech synthesis applied research.
My research interest include but not limit to Speech diagnostic for neurodegenerative disease, Responsible AI, and Spoken Dialogue System.
🔥 News
- 2024.07: Becoming a PhD Candidate at Centre for Language Studies, Radboud University
- 2024.05: One paper is accepted by INTERSPEECH 2024
- 2023.12: One paper is accepted by ICASSP 2024 (Oral)
- 2023.05: One paper is accepted by INTERSPEECH 2023
📖 Educations
- 2024 - *, PhD Candidate, Centre for Language Studies, Radboud University
- 2016 - 2018, M.Sc in Computer Science, Rutgers University–New Brunswick, NJ, USA.
- 2011 - 2015, B.Sc in Information and Computing Science, Beijing Jiaotong University, Beijing, China.
💻 Professional Experience
- 2024.7 -, PhD Candidate, Centre for Language Studies, Radboud University
- 2023.7 - 2023.10, Visiting Researcher, Audio Information Research(AIR) Lab, University of Rochester
- 2021 - 2023.7, Senior Researcher, AI-Generated Content (AIGC) Center, Tencent AI Lab
- 2018 - 2021, Machine Learning Engineer, 17 Education&Tech Group Inc. (IPO on NASDAQ)
- 2017 - 2018, Machine Learning Engineer Intern, Learnable.ai
📄Professional Service
Review Committee: ICASSP 2024, ISMIR 2023-2024
📝 Publications
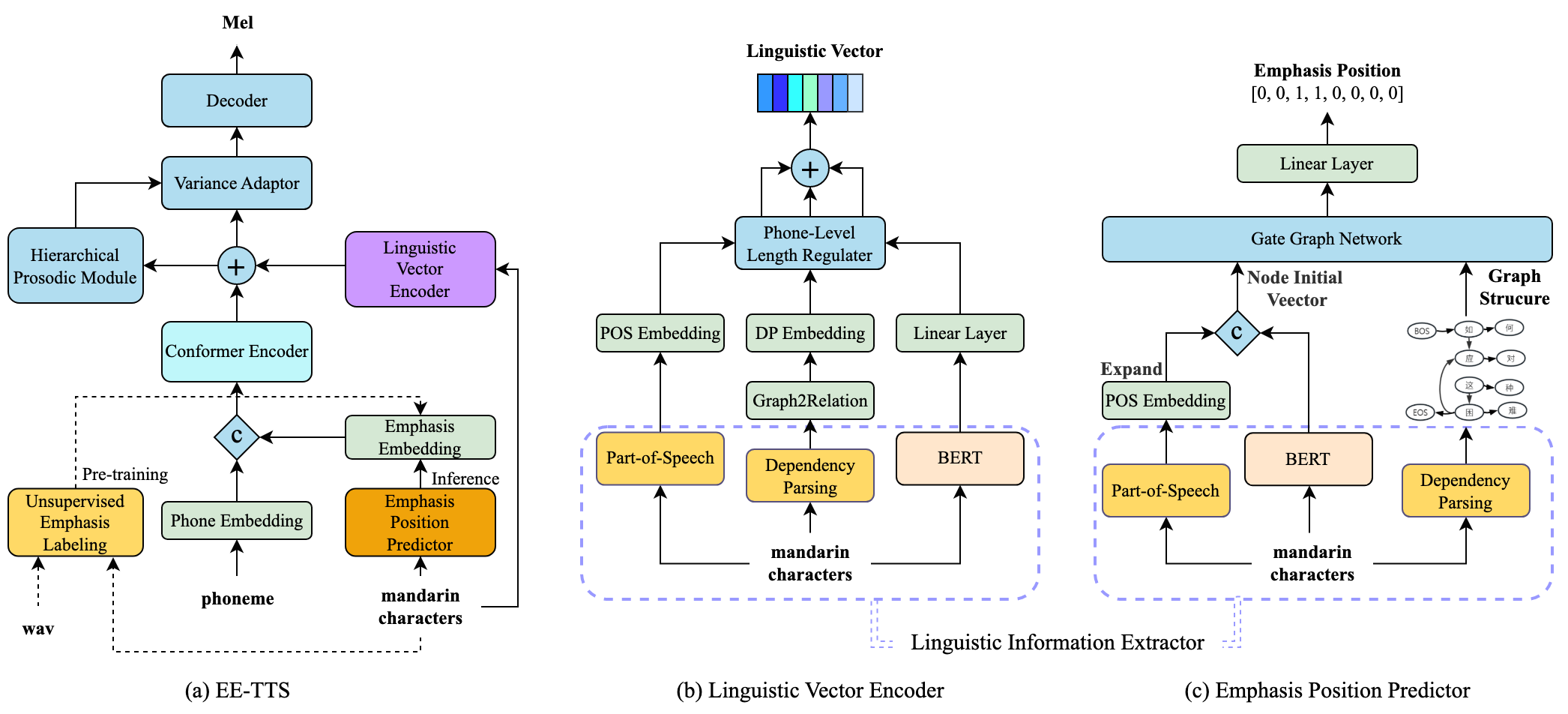
EE-TTS: Emphatic Expressive TTS with Linguistic Information
Yi Zhong, Chen Zhang, Xule Liu, Chenxi Sun, Weishan Deng, Haifeng Hu, Zhongqian Sun. [Demo Page]
Contribution:
- EE-TTS can identify appropriate emphasis positions from text and synthesize expressive speech with emphasis and linguistic information.
- This work outperforms baseline with expressiveness-MOS improvements from 3.76 to 4.25 and naturalness-MOS from 3.67 to 4.34.
- EE-TTS helps build AI playmate services for the world’s most-played mobile MOBA game Honor of Kings (DAU 100+ million).
ICASSP 2024 (Oral)
SynthTab: Leveraging Synthesized Data for Guitar Tablature Transcription
Yongyi Zang*, Yi Zhong*, Frank Cwitkowitz, Zhiyao Duan. [Demo Page]
INTERSPEECH 2024
GTR-Voice: Articulatory Phonetics Informed Controllable Expressive Speech Synthesis
Zehua Kcriss Li, Meiying Melissa Chen, *Yi Zhong*, Pinxin Liu, Zhiyao Duan. [Demo Page]
🗂️️ Selected Projects
🎙 Speech Synthesis
Few-shot Voice Cloning and Style Transfer
- Achieved few-shot voice cloning using 20 utterances. The Similarity-MOS of timbre reached 4.6 with a MOS of 3.8 and a clear pronunciation correction effect on L2 English speakers.[patented]
- Pre-train and finetune paradigm and frame-level pitch modeling are used to achieve few-shot style transfer using 20 utterances. The style SMOS has been improved from 3.5 to 4.5 while naturalness MOS remains above 4.0.
🎼 Music
Probabilistic Topic Models Based Music Recommendation System supervisor: Vladimir Pavlovic
- Leveraged CRNN for music tagging, and exploited Latent Dirichlet Allocation (LDA) and Hierarchical Dirichlet Process (HDP) probabilistic topic models for music topic modelling.
- Use KL divergence to compute the similarity of song-topic distributions for the recommendation.
💬 Speech Recognition & Evaluation
Recognition and Evaluation of Oral English
- Design and optimize the Goodness Of Pronunciation (GOP) feature, implementation, and tuning of LR, XGBoost, LSTM classifiers. Attained SOTA English oral evaluation consistency rate. [patented]
- Full pipeline chain-model training and optimization based on Kaldi framework, including corpus crawling, language and acoustic model training, Bi-RNN implementation, RNN-Rescore, etc.
- Achieved 5%-10% WER on various benchmark datasets and outperformed Google ASR API on children datasets.
🗣️ Voice Conversion
Voice Conversion Timbre Similarity Improvement
- Method: Optimized the bottleneck of hidden representation for an any-to-one PPG-pipeline VC system. [patented]
- Result: Improved the similarity MOS of voice timbre from 3.9 to 4.3.
- Implemented many-to-many VC models such as VQ-VAE, StarGAN-VC for comparison.